Introduction.
On December 20 (local time), OpenAI of the U.S. announced a new AI model, o3.
It is said to be a complex problem solver and performs well in a wide range of fields, including science, math, and coding. o3 mini will be available around the end of January 2025, followed by o3. o3 mini will be available at the end of January 2025, followed by o3. o3 mini will be available at the end of January 2025, followed by o3.
In this article, I will summarize the differences between the GPT and O series and the features of O3.
What is the O Series?
The “O Series” presented by OpenAI is a set of models that enhance the AI’s reasoning ability with a chain of thought, an approach that is different from traditional GPT models. By doing so, the ability to solve complex problems is greatly improved.
Even in the past, the GPT series prompt technique of adding “Please think step-by-step and give the correct answer” may be used for complex problems such as mathematics, and the hack-like prompt is an intrinsic part of the GPT series.
The GPT series (GPT-3 and GPT-4) learns large amounts of text data for language generation and question answering. However, GPT models have primarily sought to improve performance by scaling large amounts of data and computational resources. In contrast, the O series is characterized by its “thinking process” oriented design.
What is a chain of thought?
Chain of thought is a mechanism whereby AI follows human-like steps of thought when solving complex problems. For example, when solving a math problem, it does not directly give the answer, but rather derives the answer through a calculation process along the way. This increases the possibility of dealing with problems that do not exist in the training data.
Conventional GPT models are not good at comparisons such as “which is greater, 3.14 or 3.9?”, but the O-series can decompose the problem using the chain of thought and find the solution logically. This is the greatest strength of the O Series.
Why include thought steps? : Limitations of the conventional approach based on the Scaling Law
At first glance, this may appear to be inefficient, since adding the thinking step increases computation time. However, there was a problem that conventional language models faced that could not be solved simply by scaling up the model (increasing computational resources and data).
According to research on the Scaling Law (a law that describes the relationship between increasing the size of AI models (scale) and improving their performance), increasing model size, data volume, and computational resources improves performance, but the effect slows on a logarithmic scale.
This begins to make scaling up models inefficient in terms of cost. Especially for large-scale language models (LLMs), “qualitative improvements” such as chain-of-thought are essential because of the exponential need for data and computational resources.
The paper on Scaling Laws is here: Scaling Laws for Neural Language Models (arXiv)
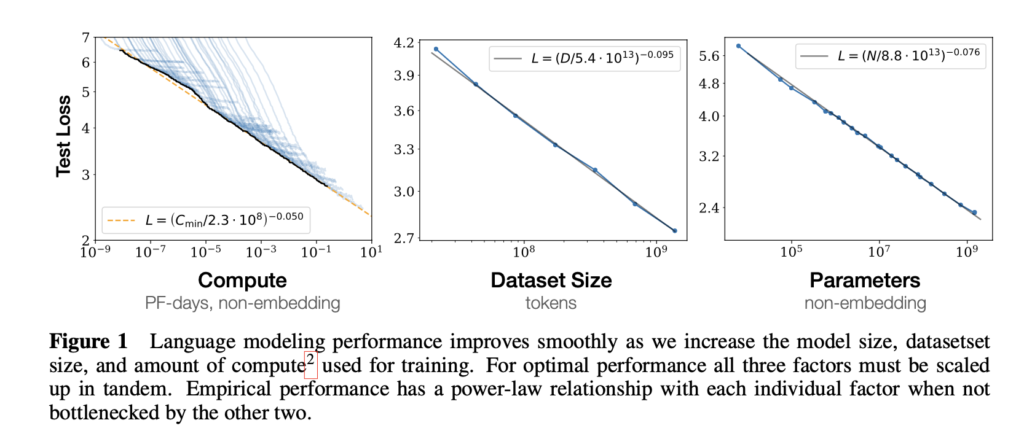
Evolution from O1 to O3
The O1 model has been shown to improve performance with different constraints than GPT; O1 generates answers to simple questions through a thought process, which significantly improves accuracy; Google’s Gemini and others have adopted similar approaches.
O3 has further evolved and dramatically improved its ability to solve complex mathematical and reasoning problems. In fact, O3 achieves 25.2% accuracy on complex mathematical reasoning problems, compared to less than 2% for traditional models, a staggering difference.
He has even placed 175th globally in a test called competitive programming, which is a test of programming algorithms within a time limit. This is a level that most people can no longer achieve.
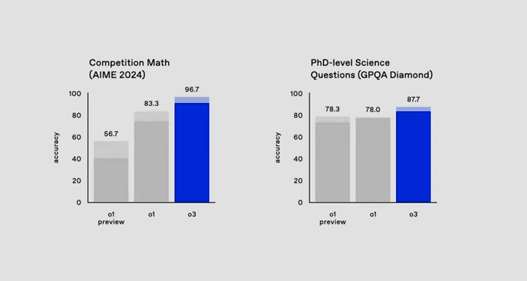
In addition, the system is now able to learn and solve on-the-fly pattern recognition problems that are easy for humans to answer but difficult for AI.
AI can also handle IQ tests and puzzle problems that often appear in job hunting.
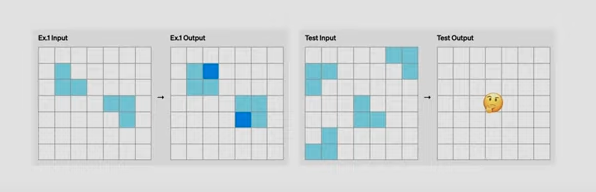
IQ tests are already coming in over 130 in O1, so I wonder what O3 will be like, maybe over 150.
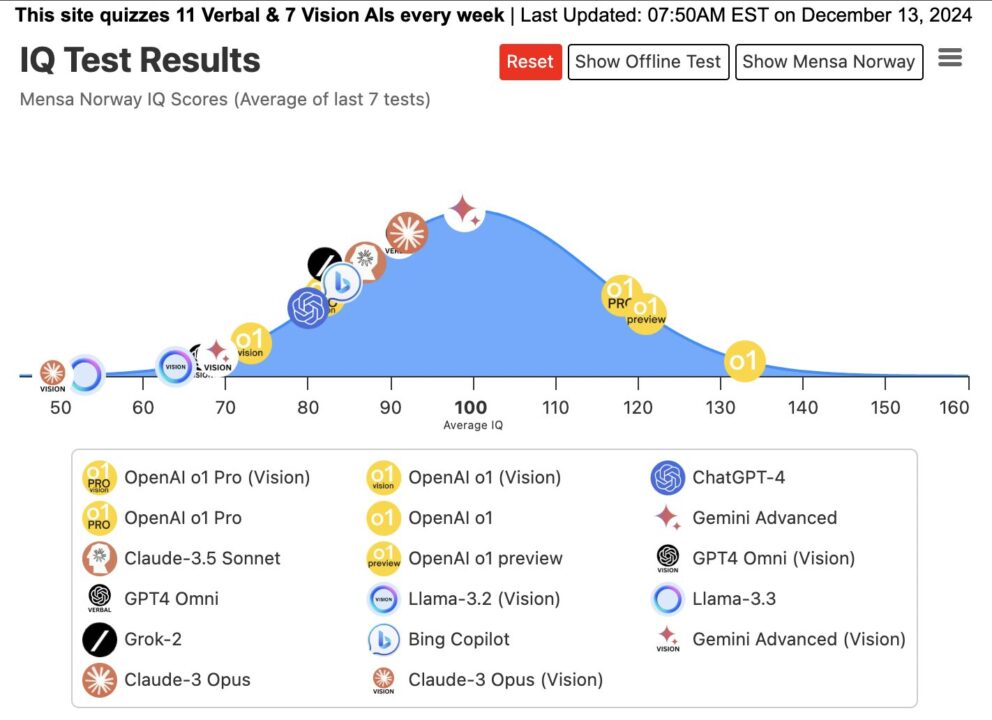
In the benchmark “Ark-AGI,” which measures how close it is to AGI (general-purpose artificial intelligence), it exceeded the human average, reaching a maximum of 87.5% (o1 reached a maximum of 32%).
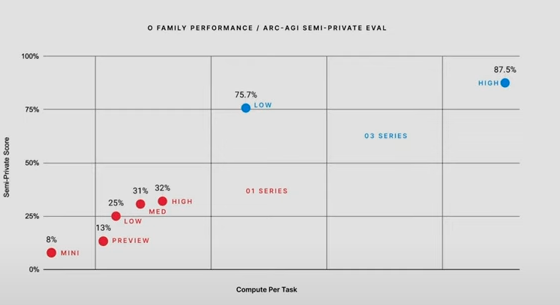
I have just released O1 and my impression is that it will still evolve from here. In the future, if we can find a method to further advance AI using approaches other than Chain of Thought (I think researchers are desperately searching and trying various approaches at the same time), it may evolve more dramatically.