- Introduction.
- What is the free energy principle?
- Prediction and Forecast Error Update Process
- Effects of noise and signal accuracy
- Caution and signal accuracy
- Examples of highly accurate sensory signals: new experiences and environments
- Example of highly accurate predictive signal: Known environment or situation
- Perception is established when the prediction error is minimized
- From sensory to motor, emotional, and psychiatric disorders
- Relationship between the brain and sleep
- Conclusion: The Future of LLM Reasoning Using Human Brain Reasoning
Introduction.
I had been interested in this book, but it had been piled up in my reading pile, so I finally finished it this time. I found the book interesting in its perspective of trying to unify seemingly disparate brain activities such as sensation, movement, emotion, and mental illness from the perspective of free energy.
A memorandum is included below.
This book, “The Grand Unified Theory of the Brain: What is the Free Energy Principle?” explains how our brains perceive, adapt, and learn about the external world from the perspective of the free energy principle. The theory is novel in that it goes beyond the framework of conventional brain science and can be applied in a unified way to sensory, motor, emotional, and even mental illness. The book also describes the cyclical mechanism of bottom-up and top-down processing, and touches on the flexible and dynamic nature of the brain.
What is the free energy principle?
The central idea of the free energy principle is that the brain adapts to its environment by minimizing free energy. This idea is based on the law of energy efficiency proposed by the 19th century physicist Helmholtz. The brain predicts external conditions and continually updates its own model by minimizing the discrepancy between its predictions and actual observations (prediction error).
This process is not just a bottom-up way of receiving and processing sensory stimuli. Rather, we believe that the brain interprets sensory input based on top-down processing, or “anticipation.”
The basic idea is that the brain does not try to understand the sensory signal itself, but rather tries to infer the cause and structure of the external environment that caused the sensory signal. This inference is based on the following elements
Prior Probability Distribution
Prior knowledge or assumption (predictive model) that the brain has about conditions in the external environment. For example, “I hear birds singing in the morning because they are nearby.
Conditional Probability Distribution (Likelihood)
The probability that a given external environmental condition will produce a particular sensory signal. For example, the association “if a bird is singing, you hear a sound of a particular frequency.
Posterior Probability
The brain’s interpretation of how the outside environment should be inferred from the current sensory signals, which is obtained by multiplying the above two pieces of information. By combining prior knowledge and the data obtained from the sensory signals, the most likely state of the environment is inferred.
Prediction and Forecast Error Update Process
Based on sensory signals and predictive models, the brain understands its environment through the following process
Generating Predictive Models
The brain uses prior and conditional probability distributions to predict sensory signals. This works as a top-down process.
Reception of sensory signals
Receives sensory signals (bottom-up processing) from the outside world. This signal may contain noise (error).
Calculation of Prediction Error
Compares the actually received sensory signal with the predicted signal and calculates the discrepancy (prediction error).
Error Correction and Model Update
Based on the prediction error, the brain modifies the prediction model. This modification is repeated, and when the prediction error is minimized, the understanding of the external environment becomes stable as a “perception.
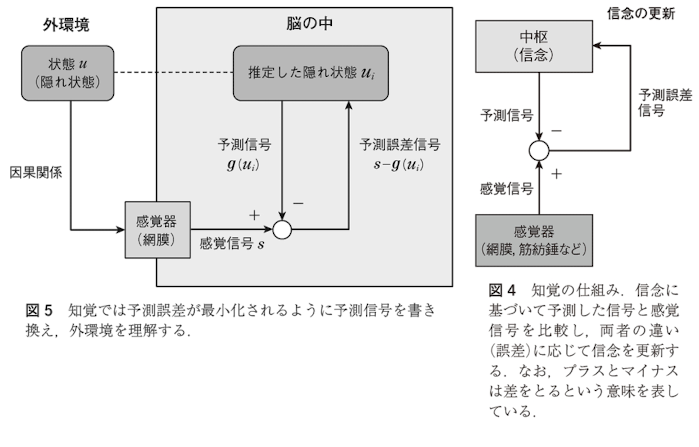
Effects of noise and signal accuracy
Both the predictive and sensory signals contain noise, which affects the prediction error. The degree to which the error is important depends on the accuracy of the signal.
When sensory signals are more accurate
When sensory signals are clear and have little noise, more weight is given to sensory signals in the calculation of prediction error. This makes the prediction model more likely to be modified based on the sensory signal.
When the accuracy of the prediction signal is high
If the prediction model is very accurate, the prediction signal is prioritized over the sensory signal, and even if the prediction error is large, the need for correction is considered low.
Caution and signal accuracy
The act of paying attention is considered a process that improves the accuracy of sensory signals. Specifically, it has the following effects.
Sensory signals to which attention is directed are processed more clearly, with noise suppressed. This improves the accuracy of the prediction error calculation.
The higher the accuracy of the sensory signal, the more accurate the correction of the prediction error. This ensures that the prediction model is properly updated for the sensory signal. On the other hand, when the accuracy of the prediction signal is high, some misalignment of the sensory signal is ignored.
This alone is difficult to understand, so I will try to describe it with concrete examples from daily life.
Examples of highly accurate sensory signals: new experiences and environments
When we see an animal or food for the first time, the brain does not have a strong predictive model about it. Therefore, it builds its understanding based on the details of the sensory signals (sight and touch). For example, when we see a dragon fruit for the first time, the brain updates its predictive model by incorporating data on its shape, color, and texture.
Example of highly accurate predictive signal: Known environment or situation
Even on rainy days when visibility is poor on a road that has been used for many years to commute to work, the brain maintains the prediction that if you turn this corner, you should be able to find the station. Even if the sensory signals are ambiguous, the accuracy of the prediction model is so high that deviations in the sensory signals are not taken into account. Thus, even if the station is actually under construction and looks slightly different, the brain judges that the station is there.
Perception is established when the prediction error is minimized
Finally, we “perceive” the state of the external environment when the prediction model is in perfect agreement with the sensory signals and the prediction error approaches zero. Perception is not simply the act of receiving the sensory signal itself, but is established when the brain infers the cause and structure of the external environment, and when that inference matches the sensory signal, according to the book.
The point that the free energy principle indicates that “perception is established at the stage where prediction error is minimized” seems to go against common intuition, but this point is a novelty. Human senses tend to perceive that “what we see with our eyes is transmitted directly to our brain in real time and forms an image immediately,” but this “real-time nature” is the result of the brain’s extremely fast and efficient process of minimizing prediction error, according to the theory proposed in the book.
There is a delay of several tens of milliseconds before visual information reaches the brain and is processed, and it is thought that during this delay the brain makes predictions and error corrections.
From sensory to motor, emotional, and psychiatric disorders
What makes the free energy principle particularly interesting is its wide range of application. In this book, the theory provides a unified explanation not only of sensory processing, but also of movement, emotion, and even mental illness.
For example, movement is interpreted as the “realization of a prediction. The brain predicts a particular action and the body moves to realize that prediction. Emotion, on the other hand, is explained as the processing of prediction errors based on changes in visceral sensation, providing a new perspective on traditional emotion theory.
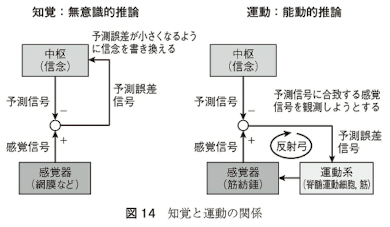
He also states that psychiatric disorders such as schizophrenia and autism can be understood as abnormalities in the processing of prediction errors. In schizophrenia, the prediction model is rigid, and in autism, the prediction model is considered overly rigid.
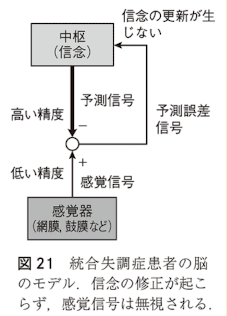
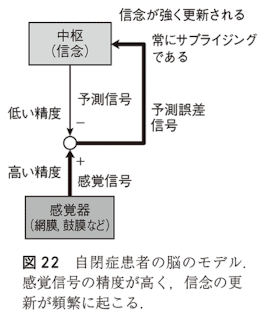
Because schizophrenia does not adequately suppress sensory signals, sensory signals become more dominant than predictive signals, resulting in less correction of the prediction error signal.
The result is hallucinatory hallucinations in which one’s own internal thoughts are perceived as “external voices,” and delusions and distorted perceptions that deviate from the reality of the situation as a result of the brain’s reliance solely on prior beliefs.
Relationship between the brain and sleep
The role of sleep is critical in the process by which the brain continually updates its predictive models. This book explains that synaptic pruning occurs during sleep. In this process, unnecessary synapses accumulated during daytime activities are organized and only important information is retained. As a result, the brain’s predictive model is reset and efficiency is improved.
While awake, they try to build accurate models by having various experiences, but this process leads to overly complex models, so they perform synaptic pruning during sleep to simplify their models.
Conclusion: The Future of LLM Reasoning Using Human Brain Reasoning
This is not mentioned in the book and is my own guess, but the LLM at the moment basically separates “learning” from “inference” and the inference done in the LLM is different in meaning from the brain inference described in the book (= always making predictions about the external world and minimizing prediction errors with actual data)
Current LLMs are trained on large amounts of data in advance and use a fixed model during inference, and new experience cannot be incorporated into the model during inference (regular LLMs require additional re-training). If the model detects errors during inference, the errors cannot be immediately reflected in learning (model updating).
We felt that LLM could be further developed by incorporating this free energy theory into LLM and advancing LLM to update the model dynamically in real time (=inference).
With the ability to perform real-time inference, LLMs will evolve from the current “fixed knowledge-based response” to “real-time intelligence” with dynamic and adaptive interactions, and will be able to do the following
- Individualized learning assistant: AI analyzes learner responses (facial expressions, tone of voice, and answers) in real time, detects areas of understanding or confusion, and changes explanations or adjusts question difficulty on the spot.
- Medical Diagnosis Assistant: AI monitors patient-physician interaction in real time and evolves the diagnosis by continuously reflecting new information (patient statements, facial expressions, updated test results).
- Personal assistant: The assistant detects stress and emotions in real time based on the user’s tone of voice, speed, and keyboard input delay, and responds appropriately.